Alternative data pricing trends 3Q22
Daryl Smith, CFA, Head of Research (London)
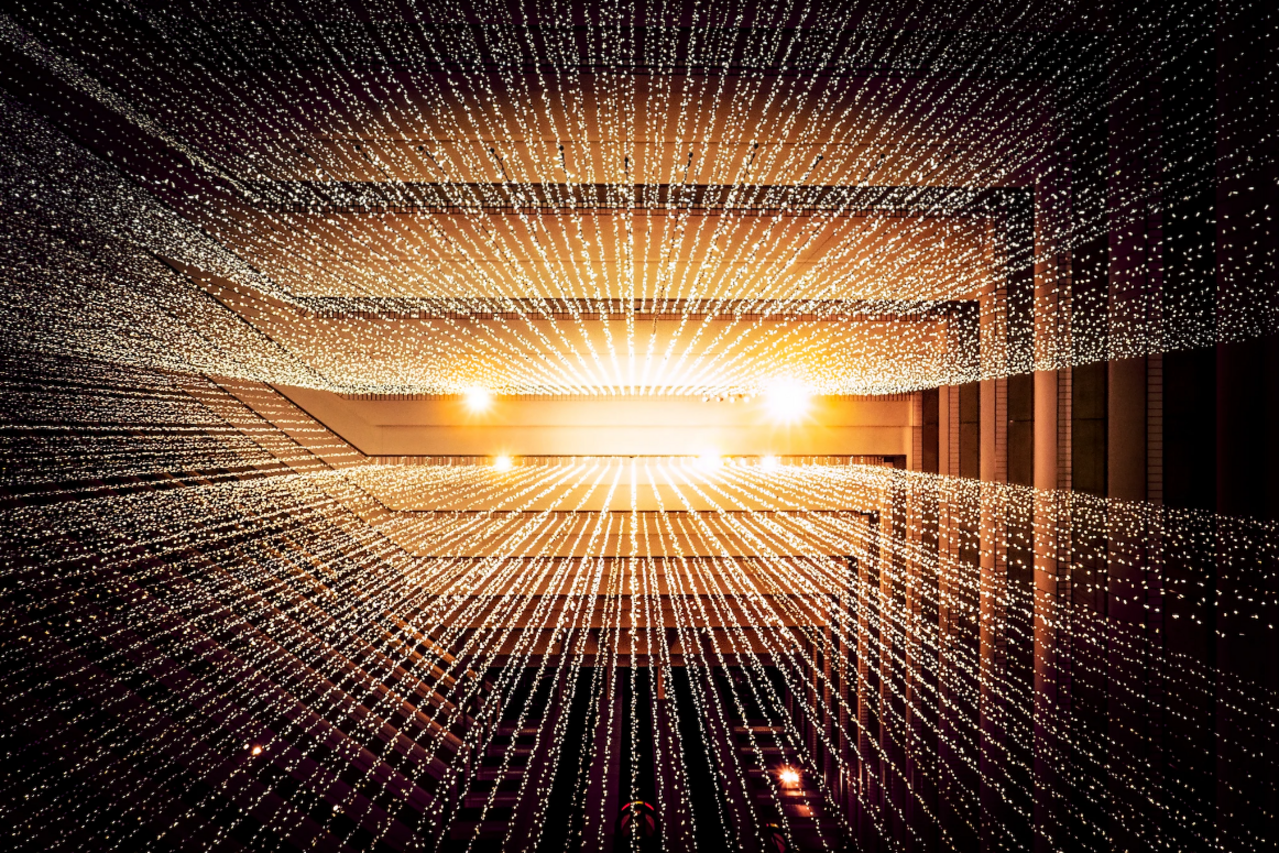
How much does alternative data cost? In our Getting the Price Right series, we leverage our unique position in the industry and proprietary data on pricing to shed some light on this topic. This 3Q22 update of our 2Q20 and 2Q19 analysis provides fresh insight into the state of alternative data pricing.