Hunting unicorns: Using alternative data to predict startup success
Paris Tung, Associate (London)
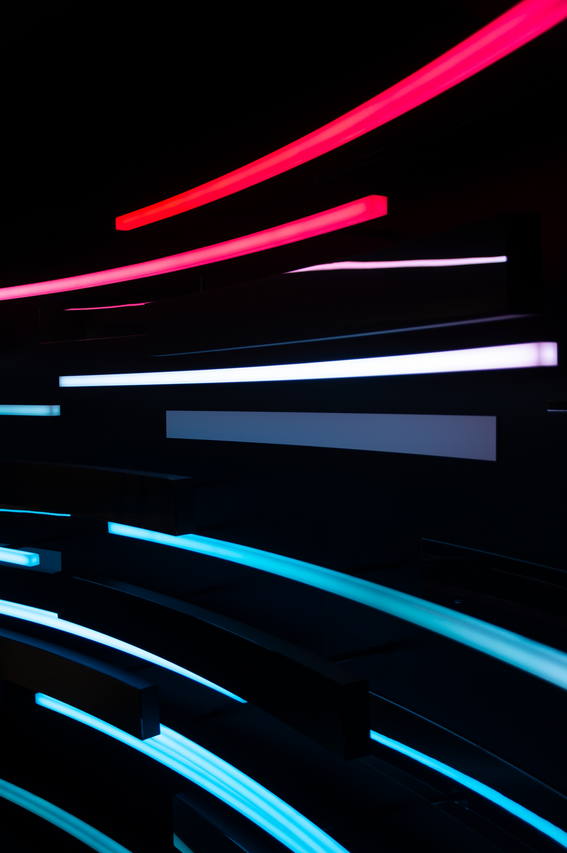
Artificial intelligence has changed the game when it comes to identifying high-potential startups. Deep learning has created new ways for venture capital firms to exploit traditional and alternative data for sourcing investment opportunities. In this literature review, we summarise a study that distils existing industry knowledge on the subject.
QUICK VIEW
- The paper discusses how deep-learning techniques are deployed in the deal-sourcing stage of investments. At first, the paper touches on ways to train models on a range of alternative data to identify startups that will be successful.
- The authors identify and discuss nine key tasks to the deep-learning life cycle: problem scoping, success definition, data gathering, data processing, data split, model selection, model evaluation, model explanation and model productisation.
- The paper conducts a meta-analysis of past literature that discusses the use of deep learning to predict startup success.
- Deep-learning algorithms can be trained on topics like company funding, product/services, meta info, founders/owners, teams, investors, web, competitors, operations, IP and R&D, customers, financials and M&A.