London Summit 2024: Artificial intelligence
Sondra Campanelli, Head of News and Marketing (London)
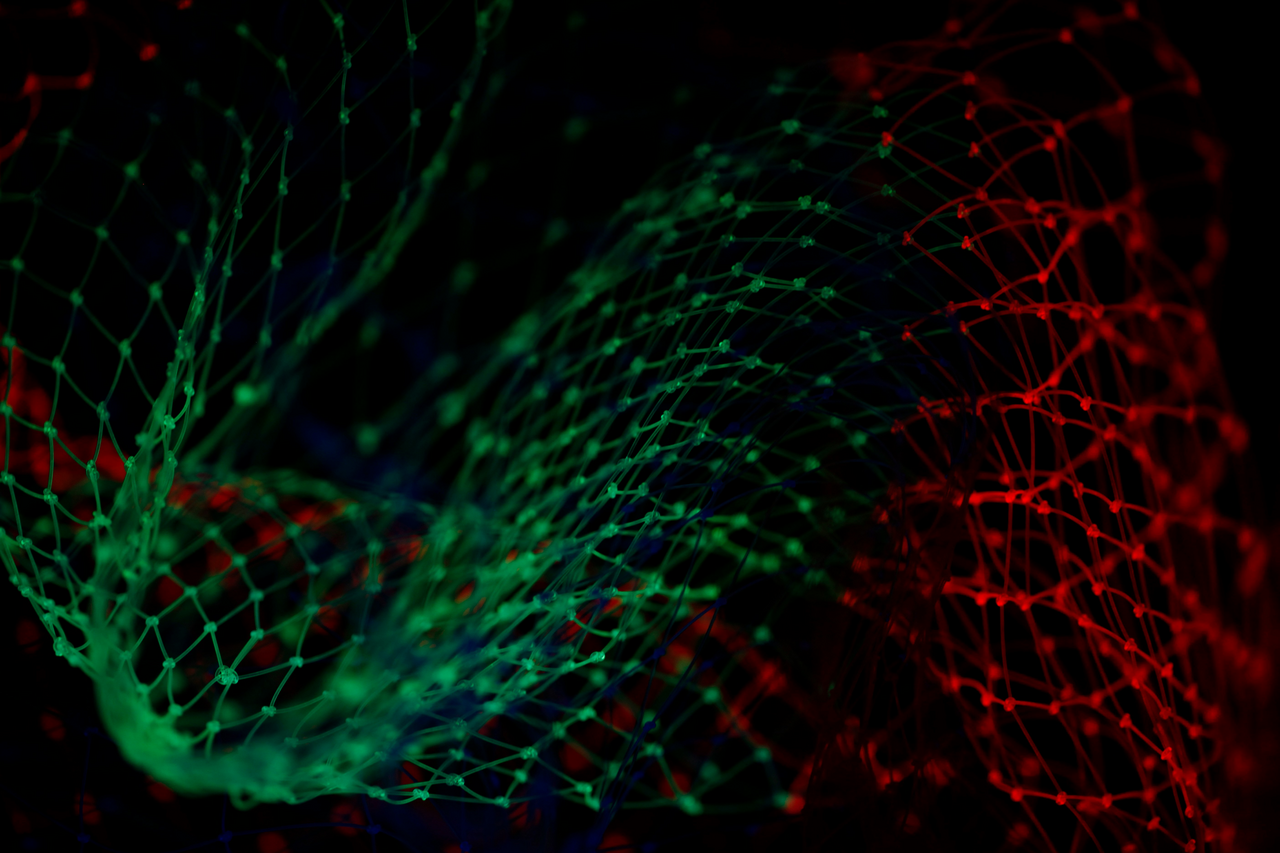
While the topic of artificial intelligence came up in most sessions at our London summit, it was often met with a weary sigh. One panellist bemoaned the “nauseating buzzword bingo around GenAI”.
The message from data buyers at the summit was clear: vendors should deploy AI with caution. “The more processed data is, the less useful it is,” said Sanne de Boer, co-head of quantitative equities at Voya IM, during a session on alt data for discretionary investors. “We like to control that ourselves.”
Indeed, Voya has already rolled out a suite of its own AI-powered portfolio managers. Its flagship AI strategy will celebrate its fourth anniversary this year and has been in development for even longer than that – over a decade, in fact.
However, the speed of development in GenAI prompted one speaker at the summit to wonder if tomorrow’s AI analysts can be developed much quicker. To test this, Chris Kantos, head of quantitative research at Alexandria Technology, has been using large language models (LLMs) to perform sentiment analysis on earnings calls.
He described his experiments in a session titled: Is ChatGPT a good stock picker? The short answer is no. “Out of the box, it’s not something I’d turn my investments over to tomorrow,” he said.
A more nuanced answer is… not yet. Backtested on S&P 500 stocks, ChatGPT out-performed generic natural-language processing (NLP) techniques such as sentiment dictionaries and transformers, but was comfortably out-matched by Alexandria’s own machine-learning ensemble model.
Even so, Kantos sees potential in fine-tuning LLMs with some specialist training to help them do a better job of analysing earnings reports. “I’m excited about the opportunity ahead,” he said.
The bar is high for using GenAI to produce investment insights, but several investors said they use machine learning heavily in upstream processes such as tagging and cleaning raw data and summarising content.
Whether it makes sense to build such AI solutions in-house was the topic of another session.
“You need to ask whether the process is core to how you make money?” said Alberto Lopez Rueda, a former data scientist at JP Morgan. “If it’s your secret sauce, you’ll want to keep it close to your chest.”
Another consideration is how generic the need is. For a use case with a big addressable market, there’s a good chance that your in-house solution won’t have much longevity.
“Someone will probably come along and build a better solution within six months,” said Lopez.
Failing that, the pace of change means that in-house solutions can either have high maintenance costs to stay current or can quickly become outdated.
Investment firms need to carefully weigh all these considerations before deciding to build their own AI solutions. In many cases, it may make sense to buy.
Buzzwords aside, GenAI and other advanced machine-learning techniques are set to continue shaping the alt data landscape.